一、项目需求
使用Scrapy爬取链家网中苏州市二手房交易数据并保存于CSV文件中
要求:
房屋面积、总价和单价只需要具体的数字,不需要单位名称。
删除字段不全的房屋数据,如有的房屋朝向会显示“暂无数据”,应该剔除。
保存到CSV文件中的数据,字段要按照如下顺序排列:房屋名称,房屋户型,建筑面积,房屋朝向,装修情况,有无电梯,房屋总价,房屋单价,房屋产权。
二、项目分析
流程图
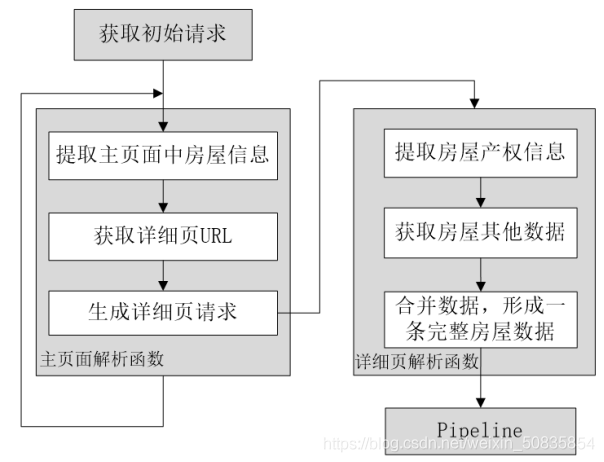
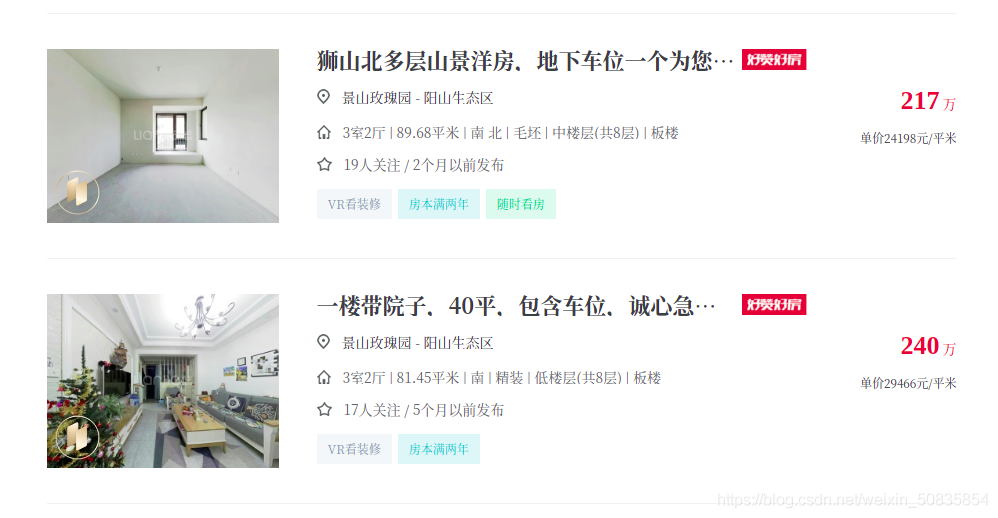
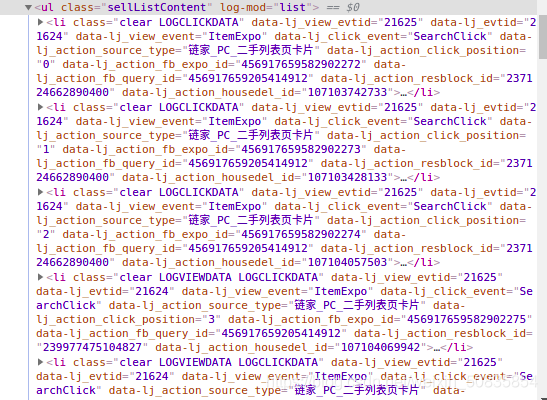
通过控制台发现所有房屋信息都在一个ul中其中每一个li里存储一个房屋的信息。

找了到需要的字段,这里以房屋名称为例,博主用linux截图,没法对图片进行标注,这一段就是最中间的“景山玫瑰园” 。
其他字段类似不再一一列举。
获取了需要的数据后发现没有电梯的配备情况,所以需要到详细页也就是点击标题后进入的页面,
点击标题
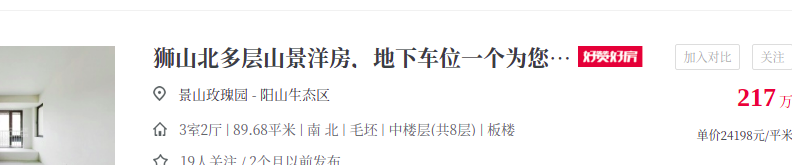
可以看到里面有下需要的信息。

抓取详细页url
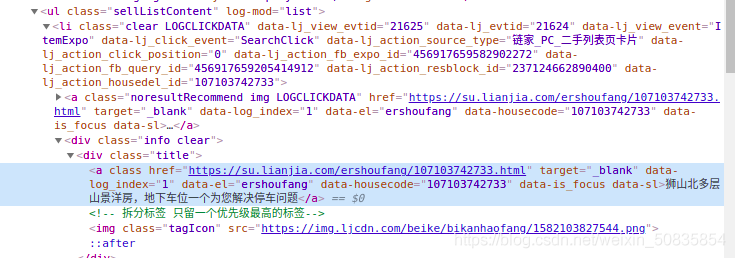
进行详细页数据分析
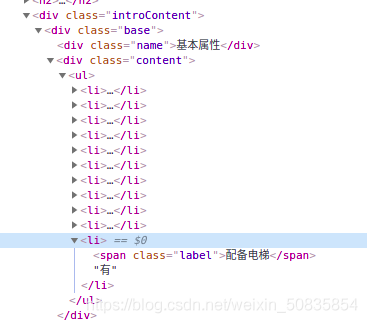
找到相应的位置,进行抓取数据。
三、编写程序
创建项目,不说了。
1.编写item(数据存储)
import scrapy
class LianjiaHomeItem(scrapy.Item):
name = scrapy.Field() # 名称
type = scrapy.Field() # 户型
area = scrapy.Field() # 面积
direction = scrapy.Field() #朝向
fitment = scrapy.Field() # 装修情况
elevator = scrapy.Field() # 有无电梯
total_price = scrapy.Field() # 总价
unit_price = scrapy.Field() # 单价
2.编写spider(数据抓取)
from scrapy import Request
from scrapy.spiders import Spider
from lianjia_home.items import LianjiaHomeItem
class HomeSpider(Spider):
name = "home"
current_page=1 #起始页
def start_requests(self): #初始请求
url="https://su.lianjia.com/ershoufang/"
yield Request(url=url)
def parse(self, response): #解析函数
list_selctor=response.xpath("//li/div[@class='info clear']")
for one_selector in list_selctor:
try:
#房屋名称
name=one_selector.xpath("//div[@class='flood']/div[@class='positionInfo']/a/text()").extract_first()
#其他信息
other=one_selector.xpath("//div[@class='address']/div[@class='houseInfo']/text()").extract_first()
other_list=other.split("|")
type=other_list[0].strip(" ")#户型
area = other_list[1].strip(" ") #面积
direction=other_list[2].strip(" ") #朝向
fitment=other_list[3].strip(" ") #装修
price_list=one_selector.xpath("div[@class='priceInfo']//span/text()")
# 总价
total_price=price_list[0].extract()
# 单价
unit_price=price_list[1].extract()
item=LianjiaHomeItem()
item["name"]=name.strip(" ")
item["type"]=type
item["area"] = area
item["direction"] = direction
item["fitment"] = fitment
item["total_price"] = total_price
item["unit_price"] = unit_price
#生成详细页
url = one_selector.xpath("div[@class='title']/a/@href").extract_first()
yield Request(url=url,
meta={"item":item}, #把item作为数据v传递
callback=self.property_parse) #爬取详细页
except:
print("error")
#获取下一页
self.current_page+=1
if self.current_page=100:
next_url="https://su.lianjia.com/ershoufang/pg%d"%self.current_page
yield Request(url=next_url)
def property_parse(self,response):#详细页
#配备电梯
elevator=response.xpath("//div[@class='base']/div[@class='content']/ul/li[last()]/text()").extract_first()
item=response.meta["item"]
item["elevator"]=elevator
yield item
3.编写pipelines(数据处理)
import re
from scrapy.exceptions import DropItem
class LianjiaHomePipeline:#数据的清洗
def process_item(self, item, spider):
#面积
item["area"]=re.findall("\d+\.?\d*",item["area"])[0] #提取数字并存储
#单价
item["unit_price"] = re.findall("\d+\.?\d*", item["unit_price"])[0] #提取数字并存储
#如果有不完全的数据,则抛弃
if item["direction"] =="暂无数据":
raise DropItem("无数据,抛弃:%s"%item)
return item
class CSVPipeline(object):
file=None
index=0 #csv文件行数判断
def open_spider(self,spider): #爬虫开始前,打开csv文件
self.file=open("home.csv","a",encoding="utf=8")
def process_item(self, item, spider):#按要求存储文件。
if self.index ==0:
column_name="name,type,area,direction,fitment,elevator,total_price,unit_price\n"
self.file.write(column_name)#插入第一行的索引信息
self.index=1
home_str=item["name"]+","+item["type"]+","+item["area"]+","+item["direction"]+","+item["fitment"]+","+item["elevator"]+","+item["total_price"]+","+item["unit_price"]+"\n"
self.file.write(home_str) #插入获取的信息
return item
def close_soider(self,spider):#爬虫结束后关闭csv
self.file.close()
4.编写settings(爬虫设置)
这里只写下需要修改的地方
USER_AGENT = 'Mozilla/5.0 (X11; Linux x86_64) AppleWebKit/537.36 (KHTML, like Gecko) Chrome/90.0.4430.72 Safari/537.36'
#为装成浏览器
ROBOTSTXT_OBEY = False #不遵循robots协议
ITEM_PIPELINES = {
'lianjia_home.pipelines.LianjiaHomePipeline': 300,
#先进行数字提取
'lianjia_home.pipelines.CSVPipeline': 400
#在进行数据的储存
#执行顺序由后边的数字决定
}
这些内容在settings有些是默认关闭的,把用来注释的 # 去掉即可开启。
5.编写start(代替命令行)
from scrapy import cmdline
cmdline.execute("scrapy crawl home" .split())
附上两张结果图。
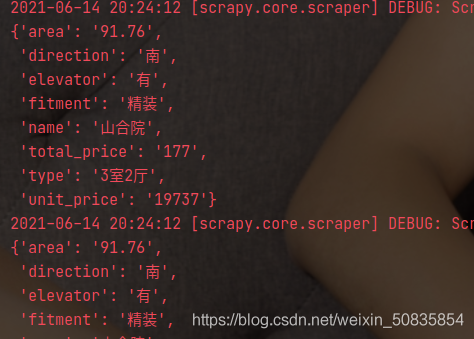
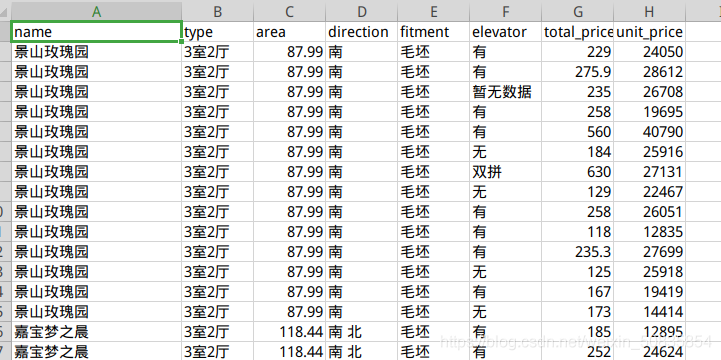
总结
此次项目新增了简单的数据清洗,在整体的数据抓取上没有增加新的难度。
到此这篇关于Python scrapy爬取苏州二手房交易数据的文章就介绍到这了,更多相关scrapy爬取二手房交易数据内容请搜索脚本之家以前的文章或继续浏览下面的相关文章希望大家以后多多支持脚本之家!
您可能感兴趣的文章:- Python手拉手教你爬取贝壳房源数据的实战教程
- Python爬虫之爬取我爱我家二手房数据
- python爬取链家二手房的数据
- Python爬虫之爬取二手房信息
- 基于python爬取链家二手房信息代码示例
- python爬虫 爬取58同城上所有城市的租房信息详解
- Python爬虫入门案例之爬取二手房源数据