目录
- 前言
- 一、动态验证码
- 二、滑动验证码
- 三、验证码的降噪
- 四、验证码的识别
- 总结
前言
python对动态验证码、滑动验证码的降噪和识别,在各种自动化操作中,我们经常要遇到沿跳过验证码的操作,而对于验证码的降噪和识别,的确困然了很多的人。这里我们就详细讲解一下不同验证码的降噪和识别。
一、动态验证码
- 动态验证码是服务端生成的,点击一次,就会更换一次,这就会造成很多人在识别的时候,会发现验证码一直过期
- 这是因为,如果你是把图片下载下来,进行识别的话,其实在下载的这个请求中,其实相当于点击了一次,这个验证码的内容已经被更换了
- 最好的方法是,打开这个页面后,将页面进行截图,然后定位到验证码的位置,将验证码从截图上面裁剪下来进行识别,这样就不会造成多次请求,验证码更换的情况了
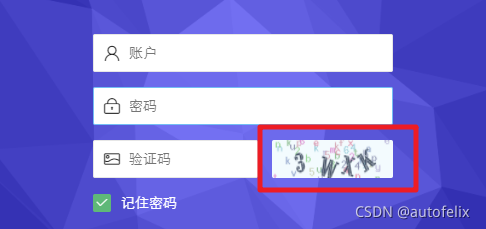
from selenium import webdriver
from PIL import Image
# 实例化浏览器
driver = webdriver.Chrome()
# 最大化窗口
driver.maximize_window()
# 打开登陆页面
driver.get(# 你的url地址)
# 保存页面截图
driver.get_screenshot_as_file('./screen.png')
# 定位验证码的位置
location = driver.find_element_by_id('login_yzm_img').location
size = driver.find_element_by_id('login_yzm_img').size
left = location['x']
top = location['y']
right = location['x'] + size['width']
bottom = location['y'] + size['height']
# 裁剪保存
img = Image.open('./screen.png').crop((left,top,right,bottom))
img.save('./code.png')
driver.quit()
二、滑动验证码
- 滑动验证码,通常是两个滑块图片,将小图片滑动到大图片上的缺口位置,进行重合,即可通过验证
- 对于滑动验证码,我们就要识别大图上面的缺口位置,然后让小滑块滑动响应的位置距离,即可
- 而为了让你滑动起来,更加的拟人化,你需要一个滑动的路径,模拟人为去滑动,而不是机器去滑动
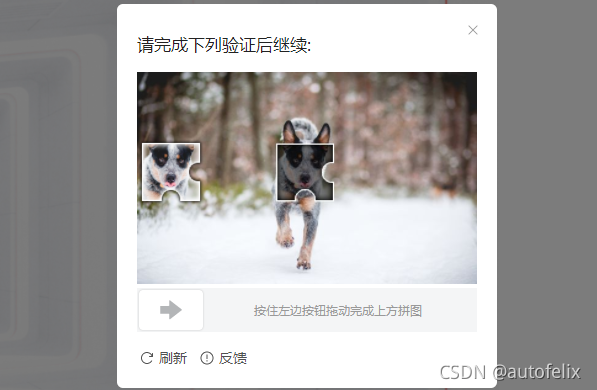
# 下载两个滑块
bg = self.driver.find_element_by_xpath('//*[@id="captcha_container"]/div/div[2]/img[1]').get_attribute('src')
slider = self.driver.find_element_by_xpath('//*[@id="captcha_container"]/div/div[2]/img[2]').get_attribute('src')
request.urlretrieve(bg, os.getcwd() + '/bg.png')
request.urlretrieve(slider, os.getcwd() + '/slider.png')
# 获取两个滑块偏移量方法
def getGap(self, sliderImage, bgImage):
'''
Get the gap distance
:param sliderImage: the image of slider
:param bgImage: the image of background
:return: int
'''
bgImageInfo = cv2.imread(bgImage, 0)
bgWidth, bgHeight = bgImageInfo.shape[::-1]
bgRgb = cv2.imread(bgImage)
bgGray = cv2.cvtColor(bgRgb, cv2.COLOR_BGR2GRAY)
slider = cv2.imread(sliderImage, 0)
sliderWidth, sliderHeight = slider.shape[::-1]
res = cv2.matchTemplate(bgGray, slider, cv2.TM_CCOEFF)
a, b, c, d = cv2.minMaxLoc(res)
# print(a,b,c,d)
# 正常如下即可
# return c[0] if abs(a) >= abs(b) else d[0]
# 但是头条显示验证码的框跟验证码本身的像素不一致,所以需要根据比例计算
if abs(a) >= abs(b):
return c[0] * bgWidth / (bgWidth - sliderWidth)
else:
return d[0] * bgWidth / (bgWidth - sliderWidth)
# 移动路径方法
def getTrack(self, distance):
'''
Get the track by the distance
:param distance: the distance of gap
:return: list
'''
# 移动轨迹
track = []
# 当前位移
current = 0
# 减速阈值
mid = distance * 4 / 5
# 计算间隔
t = 0.2
# 初速度
v = 0
while current distance:
if current mid:
# 加速度为正2
a = 2
else:
# 加速度为负3
a = -3
# 初速度v0
v0 = v
# 当前速度v = v0 + at
v = v0 + a * t
# 移动距离x = v0t + 1/2 * a * t^2
move = v0 * t + 1 / 2 * a * t * t
# 当前位移
current += move
# 加入轨迹
track.append(round(move))
return track
# 滑动到缺口位置
def moveToGap(self, track):
'''
Drag the mouse to gap
:param track: the track of mouse
:return: None
'''
ActionChains(self.driver).click_and_hold(self.driver.find_element_by_xpath('//*[@id="captcha_container"]/div/div[3]/div[2]/div[2]/div')).perform()
while track:
x = random.choice(track)
ActionChains(self.driver).move_by_offset(xoffset=x, yoffset=0).perform()
track.remove(x)
time.sleep(0.5)
ActionChains(self.driver).release().perform()
三、验证码的降噪
验证码的降噪,只是为了处理验证码图像上的多余的线条和干扰线,让你后期识别更加的准确,提高识别的准确度
第一步:可以进行灰度转化
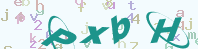
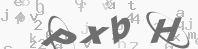
img = cv2.imread('yzm.png')
# 将图片灰度化处理,降维,加权进行灰度化c
gray = cv2.cvtColor(img,cv2.COLOR_BGR2GRAY)
cv2.imshow('min_gray',gray)
cv2.waitKey(0)
cv2.destroyAllWindows()
第二步: 二值化处理
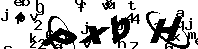
import cv2
img = cv2.imread('yzm.png')
# 将图片灰度化处理,降维,加权进行灰度化c
gray = cv2.cvtColor(img,cv2.COLOR_BGR2GRAY)
t,gray2 = cv2.threshold(gray,220,255,cv2.THRESH_BINARY)
cv2.imshow('threshold',gray2)
cv2.waitKey(0)
cv2.destroyAllWindows()
第三步:噪点过滤
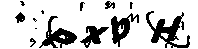
import cv2
img = cv2.imread('yzm.png')
# 将图片灰度化处理,降维,加权进行灰度化c
gray = cv2.cvtColor(img,cv2.COLOR_BGR2GRAY)
t,gray2 = cv2.threshold(gray,220,255,cv2.THRESH_BINARY)
def remove_noise(img, k=4):
img2 = img.copy()
# img处理数据,k过滤条件
w, h = img2.shape
def get_neighbors(img3, r, c):
count = 0
for i in [r - 1, r, r + 1]:
for j in [c - 1, c, c + 1]:
if img3[i, j] > 10: # 纯白色
count += 1
return count
# 两层for循环判断所有的点
for x in range(w):
for y in range(h):
if x == 0 or y == 0 or x == w - 1 or y == h - 1:
img2[x, y] = 255
else:
n = get_neighbors(img2, x, y) # 获取邻居数量,纯白色的邻居
if n > k:
img2[x, y] = 255
return img2
result = remove_noise(gray2)
cv2.imshow('8neighbors', result)
cv2.waitKey(0)
cv2.destroyAllWindows()
四、验证码的识别
通常我们会使用tesserocr识别验证码,但是这个库有很大的局限性,识别率低,即时降噪效果很好,有很少的线条,也会不准确,这种识别方式并不十分推荐
所以我们一般会使用第三方的接口进行识别,比如阿里的图片识别、腾讯也都是有的
这些第三方接口需要自己接入识别接口
#识别降噪后的图片
code = tesserocr.image_to_text(nrImg)
#消除空白字符
code.strip()
#打印
print(code)
总结
到此这篇关于python自动化操作之动态验证码、滑动验证码的降噪和识别的文章就介绍到这了,更多相关python动态验证码降噪和识别内容请搜索脚本之家以前的文章或继续浏览下面的相关文章希望大家以后多多支持脚本之家!
您可能感兴趣的文章:- python验证码识别教程之灰度处理、二值化、降噪与tesserocr识别
- python验证码识别的实例详解
- Python验证码识别处理实例
- python验证码识别教程之利用滴水算法分割图片
- python简单验证码识别的实现方法
- 详解Python验证码识别
- python验证码识别教程之利用投影法、连通域法分割图片
- python爬虫之自动登录与验证码识别
- 使用python 对验证码图片进行降噪处理
- python对验证码降噪的实现示例代码